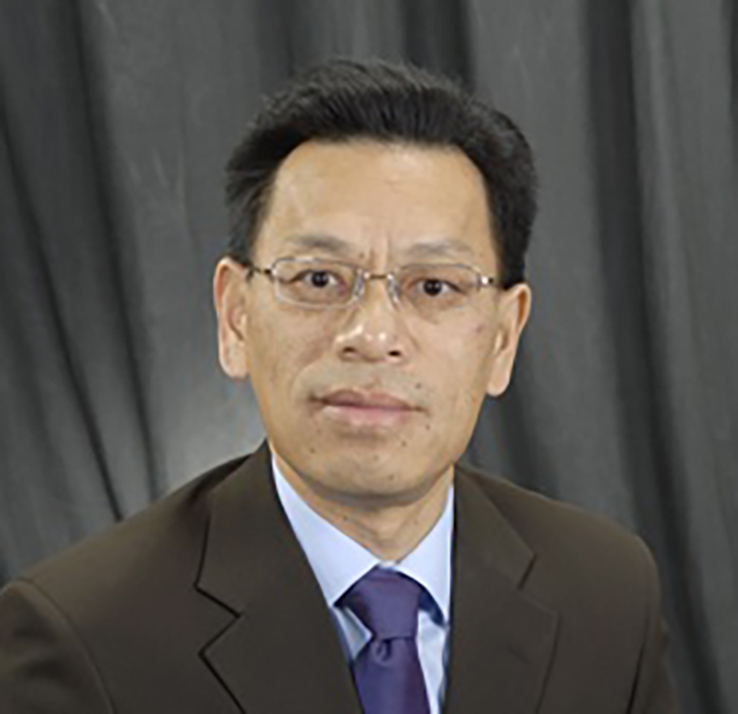
(Din)Ding-Geng Chen
University of North Carolina, Chapel Hill, USA
Title: BAYESIAN PARADIGM IN SOCIAL INTERVWENTION RESEARCH ON EVIDENCE BUILDING
Biography
Biography: (Din)Ding-Geng Chen
Abstract
Statement of the Problem: Social interventions are purposefully implemented change strategies and social intervention research is then intended to follow a design and evaluation process in which activities build on prior information over time. The process is iterative and nonlinear in refining and building new evidences. Although prior information informs successive new evidences, prior information is rarely considered in data analysis in intervention analyses. This is not consistent to our scientific principle of evidence building and new paradigm should be explored. Methodology & Theoretical Orientation: We describe a Bayesian perspective on intervention research. Bayesian methods make use of prior information in analyses. In particular, rather than ignoring prior information as in typical intervention analysis, the Bayesian approach to intervention research incorporates prior information from new data distributions based on the Bayes’ theorem. Information from prior studies can be used to formulate a posterior distribution. This posterior distribution is then incorporated in the inferential process. Therefore, a Bayesian approach to intervention research analyzes current study data by drawing on information from previous studies. The Bayesian perspective provides a sequential quantitative method for estimating outcomes in newly obtained data by making use of the previous understanding of intervention effects. Conclusion & Significance: From a research design perspective, Bayesian methods have the potential to improve power and reduce required sample sizes in intervention research. If smaller samples could be used, the cost of intervention studies might be reduced, which in turn, could reduce the design demands of intervention research.